IBM Data Governance Maturity Assessment Model
Data is considered to be the fuel that drives business growth and the engine of business innovation. Data is undoubtedly one of the assets that an organization recognizes and owns, but due to the increase in its massive data, the complexity increases, and it becomes more and more difficult to manage and control.
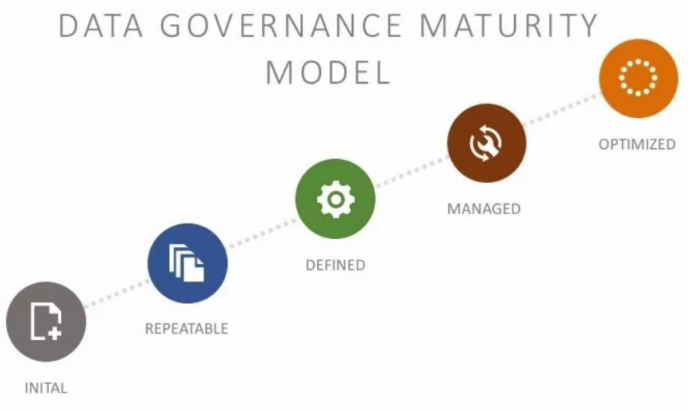
- From structured data to unstructured data. For example: customer and employee data, metadata, trade secrets, emails, video and audio, etc. Organizations need to find a proven way to manage business data needs without disrupting the normal operation of the business;
- A large amount of data is scattered in every corner. At present, the data of many organizations are distributed in multiple complex silo-type application systems. These data are isolated from each other. There is a lot of redundancy and confusion in the data. A small part of the data participates in cross-departmental collaboration. Good data governance and data management organizational structure, roles and responsibilities;
- Businesses expect to use information for performance and profit. Organizations expect to reflect the value of data by evaluating data assets, and expect to support the organization’s business decisions and reduce risks in competition through data operations.
For these reasons, data governance has been elevated to an enterprise strategic priority.
Generally, data governance is considered as the core control procedure for obtaining high-quality data, which is used to manage, use, improve and protect the data quality in the process of enterprise data processing. Effective data governance can promote cross-organizational coordination and decision-making, and enhance the availability, trust, manageability, and control of enterprise data. Four factors that businesses care about:
- increase income
- cut costs
- avoid risk
- data trustworthy
Many companies develop their own data management practices through learning, training and borrowing experience, and establish implementation methodologies by finding industry benchmarks and common frameworks. The IBM Data Governance Maturity Model is an expert committee composed of 55 experts who carry out data governance business, technology, methods and best practices through the planning, design, implementation, and verification stages, and proposes methods for obtaining consistent and high-quality data through data governance. A maturity model that helps organizations effectively improve their data management environment and make effective use of data.
The expert committee identified the main data issues facing organizations today, as follows:
- Lack of cross-functional data governance organizational structure and decision-making mechanism;
- Lack of data risk and data asset assessment resulting in a disconnect between business goals and IT;
- Lack of linkage between data governance policies and data requirements management, data analysis forecasting, and data quality reporting;
- Common data repositories, rules, standards and processes lead to data risks in data lifecycle management;
- Metadata management and business glossary, lack of data quality tracking, semantic confusion, and difficulty in quantifying business value from indicator data;
- Lack of effective data value assessment techniques and risk assessment tools to support enterprise data assessment;
- Data model management and control has long been an afterthought, lacking data architecture compliance, architecture integrity, and the effectiveness of implementation and deployment.
Maturity Level
Developed by the Software Engineering Institute (SEI) in 1984, the Capability Maturity Model (CMM) is a method for developing and improving an organization’s software development process. The CMM describes five levels of maturity paths:
Level 1. initialization
Work is often temporary and the environment is unstable, reflecting individual competencies within the organization and not being managed as maturity. Although products and services are generated in this stage, the budget and project time are often exceeded;
Level 2. managed
Effective management based on projects or single business functions, able to track costs and schedules, and reuse based on project practice planning and execution experience, but still lack overall management within the organization, and there are still risks such as budget overruns and practice overdue;
Level 3. defined
Formation of standards, processes and procedures management covering the entire organization within the organization, which can be adapted to business functions or projects within the organization;
Level 4. quantitative management
The organization conducts quantitative management of the quality objectives carried out through statistical techniques and quantitative analysis;
Level 5. continuous improvement
Quantitative objectives are clearly established and continually revised to reflect changes in business objectives.
Model Introduction
The IBM Data Governance Council proposed a data governance capability maturity model, and high-level capabilities are reflected in four aspects:
- Effectiveness
- Supporting elements
- Core principles
- Support guidelines
The promoters of the data governance maturity work are usually the information managers of the enterprise. They pay attention to the need for cross-functional, cross-process, and cross-functional boundary standardization, consider the data quality and data security requirements in the information life cycle, and focus on the organization-level data governance procedures. Carry out maturity assessment and management, and then achieve effective synergy through management.
- Data risk management, the identification, quantification, avoidance, acceptance and mitigation of risks involved in data risk management;
- Value creation, the process of evaluating and quantifying data assets, enables enterprises to maximize the value created by data assets;
- Organizational structure and culture, describe the mutual responsibility and organizational structure between business, IT, and data, and propose fiduciary responsibilities and commitments for management at different levels of the organization;
- Data management, which aims to ensure that organizations obtain high-quality data assets and carry out effective data management to reduce risks and enhance the value of data assets;
- Policy, a policy is a written statement of the behavior and norms that an organization expects to obtain in terms of data governance;
- Data quality management, providing data quality integrity, consistency, normative methods, measurement and evaluation, improvement and verification;
- Information life cycle management, life cycle-based information collection, processing, use, retention and decommissioning, etc.;
- Data security and privacy, describing the organization’s strategies, practices, and controls for risk prevention and protection of data assets;
- Data architecture, enterprise and data model management, provide complete data source access, classification, storage, circulation management, and provide available data assets and data sharing development;
- Taxonomy and metadata, for business and IT to build consistent and understandable terms, definitions, taxonomies and metadata management, data types and repository methods and tools;
- Information auditing, logging and reporting, the process of measuring and evaluating data value, risk, and governance effectiveness in terms of terms.
Evolution Route
Organizations need to confirm that there are current data problems in order to carry out effective data solutions. The best way is to obtain an evolutionary route for improving data management capabilities:
- Current Status: Where are we currently?
- Future State: Where do we want to be in the future?
- Evolution Roadmap: What people, processes, technologies, and strategies do we need to fill the gap between current and future states?
The framework of the IBM Data Governance Maturity Model provides examples of gaps between current and future states. The best way to perform a Data Governance Maturity Assessment is from a shared responsibility between business and IT.
Conclusion
Data governance has become one of the top strategic priorities for organizations around the world. Therefore, the demand for data governance across the industry continues to increase, and it is especially important to help companies obtain the current stage and positioning.
IBM data management maturity model provides a set of benchmarks and milestones, help organization measure data governance maturity, by maturity level, business practices and organizational activities and so on elements, help organizations using maturity model to carry out the overall data governance, improve the capacity to determine where we are today, where we will go to the future.